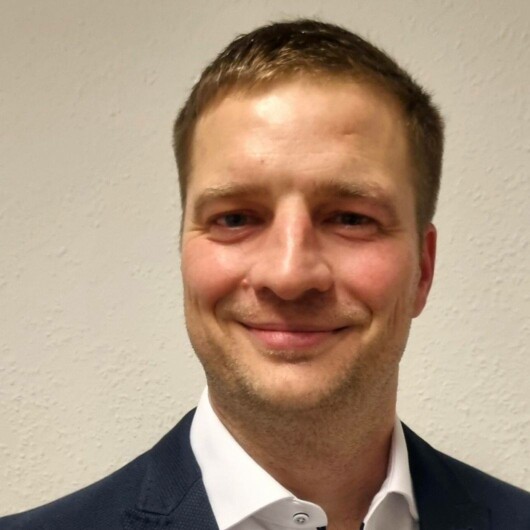
Presentation
Machine learning will change the way we work
A collaboration to use big data analysis and regression modeling to improve fab productivity and to lower manufacturing costs
Infineon views Artificial Intelligence/Machine Learning (AI/ML) as the foundation to the development of novel techniques leading to improved product performance and fab stability, whilst at the same time being a key driver of competitiveness. AI empowers the engineer to focus less on data acquisition and more on the analysis. When this is coupled with experience acquired in the fab, real opportunities to increase stability, increase yield, and lower costs become apparent to engineers in ways they did not before. AI can reduce the burden of decision-making in the fab when predicted outcomes are consistent with actual outcomes. In this context, the cost and productivity challenges posed by metrology in the fab environment clearly present an opportunity for improvement.
This presentation describes the collaboration between Infineon and Lam Research to improve costs and efficiency of the etch rate qualification and reports the results achieved.
Equipment Intelligence® data analysis (EI-DA) is a proprietary advanced multivariate big data analysis system developed by Lam Research. EI-DA polls the entire sensor matrix on Lam 2300® platforms, distilling large quantities of data through dimension reduction techniques. A novel approach, so called ‘virtual metrology’, leverages EI-DA, to seek process specific statistical parameters which best map ‘in process’ performance to on-wafer results in an accurate and repeatable way. Employing the principles of ML, the predictive models are periodically fed real on wafer data (‘y-data’), to validate the results. Through further extension of this method, Lam and Infineon have demonstrated consistent performance of the models when applied to the more cost effective ‘bare silicon’ wafers. This enables lower material cost, reduced utilization of metrology tools, and higher fab productivity.
Predictive model fitting of R²≈0.87, (RMSE≈0.5) has been demonstrated, which is consistent with real on wafer data. The model reliability offers Infineon the option to selectively replace conventional metrology operations with virtual metrology. Additionally, the method allows a metrological result to be predicted with a high degree of confidence for every wafer. Much of the decision-making process becomes automated. Solutions are arrived at earlier. Such successful applications of AI/ML enable better approaches to how we work today, and into the future, in line with the Infineon vision of “Being part of today, Being part of tomorrow”.